Datasets from the Sub-seasonal Forecast for the Low Frequency Rainfall over the Lower Reaches of the Yangtze River Valley on the Time Scale of 50 to 80 d
Yang, Q. M.
Jiangsu Meteorological Institute, Nanjing 210009, China
Abstract: The daily rainfall data (average value of 25 stations) covers the Lower Reaches of Yangtze River Valley (LRYR) during 1979-2014, along with NCEP/NCAR global 850 hPa zonal wind lattice point reanalysis data (2.5ºx2.5º), were used in this study to obtain an experimental dataset of the sub-seasonal forecasts. Then, the experimental data were used to establish an extended complex autoregressive model (ECAR) using an empirical orthogonal function (EOF) and singular spectrum analysis (SSA) method. The obtained test results of sub-seasonal daily changes in the low-frequency components of the rainfall in LRYR between 2001 and 2014 showed that the forecasted lead time of the low-frequency rainfall components in LRYR (on a time scale of 50 to 80 days) was approximately 52 days. It was observed that this study’s forecasting skills was clearly superior to that of an autoregressive model (AR) on a 50- to 80-day time scale, and the forecasting skills had reached the highest levels from June to August. This dataset included seven low-frequency components (pc1, pc2, …, pc7) in the global 850 hPa 50- to 80-day low-frequency zonal wind fields from January 1st, 1979 to December 31st, 2014, within the time series (series length of 13,149 days) of the daily low-frequency rainfall lcjr (the daily low frequency rainfall in the lower reaches of the Yangtze River Valley, lcjr) in the LRYR. This study’s dataset was archived in an excel file with the data size of 1.19 MB.
Keywords: lower reaches of the Yangtze River (LRYR); rainfall; 50-80 d low-frequency components; sub-seasonal forecasts
1 Introduction
During the past 10 years, the global data obtained by climate observations have rapidly increased. These large amounts of scientific data include high data correlations and multiple data attributes and are used to reflect and characterize complex natural phenomena and relationships[1–2]. It has been found that by extracting the effective data from the large amount of overall data, more comprehensive intra-seasonal oscillation (ISO) variation information can be obtained. This information has provided a good developmental basis for the 15- to 60-day sub-seasonal forecasts[3–5] of extreme weather events. Based on the global low-frequency circulations and low-frequency rainfall data of the Lower Reaches of the Yangtze River (LRYR), Yang established a simplified time-varying extended complex autoregressive model (ECAR)[6]. This model had the ability to obviously prolong the forecasting lead times, and displayed improved forecasting abilities for the variations of the low-frequency components of 50- to 80-day low-frequency rainfall forecasts, which were known to be significantly related to the heavy rainfall events in LRYR in future 50- to 60-day periods[7]. These sub-seasonal forecasting data were obtained from NCEP/NCAR global 850 hPa zonal wind lattice point reanalysis data (2.5°×2.5°), and the daily rainfall data in LRYR during the period ranging from 1979 to 2014 (30°30′N-32°0′N, 118°0′E-122°30′E; average value of 25 stations). Then, using band-pass filtering, empirical orthogonal function (EOF) and singular spectrum analysis (SSA) analysis, this study was able to obtain the seven low-frequency principal components (pc1, pc2, …, pc7) of the global 850 hPa 50- to 80-day low-frequency zonal wind fields from January 1st, 1979 to December 31st, 2014, as well as the time series of daily low-frequency rainfall lcjr in LRYR. A dataset of the sub-seasonal forecasts for the low-frequency rainfall over LRYR was then constructed on a time scale of 50 to 80 days.
2 Metadata of Dataset
The metadata of the dataset[8] is summarized in Table 1, including the dataset full name, short name, authors, years, data format, data size, data files, data publisher, and data sharing policy.
Table 1 Metadata summary for the datasets from the sub-seasonal forecast for the low frequency rainfall over the lower reaches of the Yangtze River Valley on the time scale of 50 to 80 d
Item
|
Description
|
Dataset full name
|
Datasets from the sub-seasonal forecast for the low frequency rainfall over the lower reaches of the Yangtze River Valley on the time scale of 50 to 80 d
|
Dataset short name
|
ForecastLowFreqRainfallLYRV
|
Authors
|
Yang. Q. M. G-9579-2018, Jiangsu Meteorological Institute, yqm0305@263.net
|
Geographic region
|
Lower Reaches of the Yangtze River: 30°30′N-32°0′N, 118°0′E-122°30′E
|
Year
|
1979 to 2014
|
Data format
|
.xls
|
Data size
|
1.19 MB
|
Dataset files
|
ForecastLowFreqRainfallLYRV.xls, daily global 850 hPa zonal wind low-frequency principal component and low-frequency rainfall on a scale of 50 to 80 days in the lower reaches of the Yangtze River from 1979 to 2014
|
Foundation(s)
|
National Natural Science Foundation of China (41175082)
|
Data publisher
|
Global change research data publishing and repository: http://www.geodoi.ac.cn
|
Address
|
No. 11A, Datun Road, Chaoyang District, Beijing 100101, China
|
Data sharing policy
|
Data from the Global Change Research Data Publishing & Repository includes metadata, datasets (data products), and publications (in this case, in the Journal of Global Change Data & Discovery). Data sharing policy includes: (1) Data are openly available and can be free downloaded via the Internet; (2) End users are encouraged to use Data subject to citation; (3) Users, who are by definition also value-added service providers, are welcome to redistribute Data subject to written permission from the GCdataPR Editorial Office and the issuance of a Data redistribution license; and (4) If Data are used to compile new datasets, the ‘ten percent principal’ should be followed such that Data records utilized should not surpass 10% of the new dataset contents, while sources should be clearly noted in suitable places in the new dataset[9]
|
3 Methodology
The 850 hPa zonal wind fields data used in this study were from the NCEP/NCAR daily reanalysis of the global wind field data (2.5°×2.5°) lattice point[10]. The total daily rainfall amounts were calculated from the average values of 25 stations in the lower reaches of the Yangtze River (30°30′N-32°0′N, 118°0′E-122°30′E). The above-mentioned data were obtained from the available data of the period ranging from January 1st, 1979 to December 31st, 2014.
3.1 Algorithm
The first seven main spatial modes (time scale: 50 to 80 days; data period: 1979 to 2000; series length: 8,036 days; the global 850 hPa low-frequency zonal wind field obtained through Butterworth filtering) were determined using the principal component analysis (PCA) of the global low-frequency zonal winds. The obtained variances were determined to be 23.5%, 4.3%, 4.1%, 3.5%, 3.0%, 2.9%, and 2.5%, respectively[7]. SSA[11] was used to conduct low-pass filtering of the original series of daily rainfall in the LRYR. Then, a time series of the main mode of the global zonal winds and the component series corresponding to the main sub-seasonal oscillation signals on a minimum time scale of one month were reconstructed. The purpose was to obtain the observed low-frequency component series of the rainfall in the LRYR, along with the low-frequency principal components which corresponded to the spatial mode of the global 850 hPa daily zonal low frequency wind fields.
3.2 Technical Process
The data of the daily observed global zonal winds from 2001 to 2014 were projected to the seven low-frequency spatial modes (series length calculated to 8,036 days according to the data from 1979 to 2000) in order to obtain the observational values of the first seven principal components (pc1-pc7), including the daily high-frequency oscillations. Then, these seven observed principal components, along with the data of the daily rainfall in the LRYR during the same period, were projected to the T-EOF which corresponded to the 50- to 80-day oscillations after SSA. The goal was to obtain the low-frequency principal components (pc1, pc2, …, pc7), as well as the 50- to 80-day reconstruction components lcjr (series length: 5,113 days) of the daily rainfall in the LRYR.
4 Results and Validation
4.1 Dataset Composition
This dataset included seven low frequency principal components (pc1, pc2, …, pc7) of the global 850 hPa 50- to 80-day low frequency zonal wind fields from January 1st, 1979 to December 31st of 2014, and the time series of the daily low-frequency rainfall lcjr in the lower reaches of the Yangtze River (series length: 13,149 days). The dataset was stored in an Excel file, and the data size was 1.19 MB.
4.2 Results
The results of this study are as follows:
(1) The seven low-frequency principal components (pc1, pc2, …, pc7) of the global 850 hPa 50- to 80-day low-frequency zonal wind fields from January 1st, 1979 to December 31st, 2014;
(2) The time series of the daily low-frequency rainfall lcjr in the lower reaches of the Yangtze River on a time scale of 50 to 80 days, from January 1st, 1979 to December 31st, 2014.
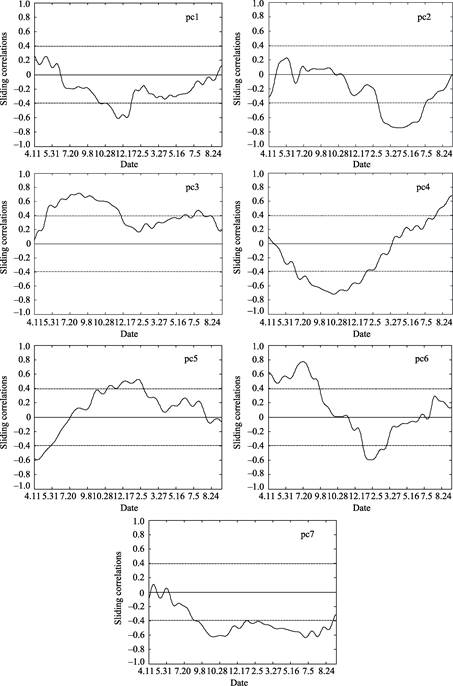
Figure 1 Sliding correlations (200 days) between the 50- to 80-day low-frequency rainfalls in the Lower Reaches of the Yangtze River from 2013 to 2014; and the principal components (pc1-pc7) of the global 850 hPa low-frequency zonal winds
4.3 Data Validation
A sliding modeling method was adopted to maintain the significant correlations between the low-frequency rainfall lcjr in LRYR and each period of the seven principal coefficients (pc1, pc2, ..., pc7) as far as possible. Then, the observed data were gradually adapted in order to obtain the main delay-dependent changes, and improve the forecasting ability of the ECAR[6]. The real-time forecasting process adopted a limited memory method to maintain the subsequent unchanged numbers. Also, the extended data matrix F=(pc1 pc2 ... pc7, lcjr) was used to establish the ECAR containing the time-varying coefficients, and conduct independent sample forecasting tests of the sliding of each data component in the extended data matrix.
5 Discussion and Conclusion
The observational data is normally characterized by multivariable properties. The dynamic data can be used to determine complicated low-frequency variation processes and systems and establish simplified sub-seasonal forecasting models. This study developed lcjr datasets for the seven low-frequency principal components (pc1, pc2, …, pc7) of the global 850 hPa 50- to 80-day low-frequency zonal wind fields, and daily low-frequency rainfall events in the Lower Reaches of the Yangtze River, in order to establish an ECAR forecasting model containing time-varying coefficients. It should be noted that the obtained 50- to 80-day low-frequency oscillation intensities displayed irregular time variations. Therefore, the follow-up research needs to extend the time-series, as well as update it over time.
References
[1] Sue, N. Big data: the Harvard computers [J]. Nature, 2008, 455: 36–37.
[2] Overpeck, J. T., Meehl, G. A., Bony, S., et al. Dealing with data: Climate data challenges in the 21st century [J]. Science, 2011, 331: 700–702.
[3] Brunet, G., Shapiro. M., Hoskins, B., et al. Collaboration of the weather and climate communities to advance subseasonal-to-seasonal prediction [J]. Bulletin of the American Meteorological Society, 2010, 91: 1397–1406.
[4] Kondrashov, D., Chekroun, M. D., Robertson, A. W., et al. Low-order stochastic model and “past-noise forecasting” of the Madden–Julian Oscillation [J]. Geophysical Research Letters, 2013, 40: 5305–5310.
[5] Chen, N., Majda, A. J. Predicting the real-time multivariate madden–Julian Oscillation Index through a low-order nonlinear stochastic model [J]. Monthly Weather Review, 2015, 143: 2148–2169.
[6] Yang, Q. M. Extended complex autoregressive model of low-frequency rainfalls over the lower reaches of Yangtze river valley for extended range forecast in 2013 [J]. Acta Physica Sinica, 2014, 63(19): 455-465. DOI: 10.7498/aps.63.199202.
[7] Yang, Q. M. A study on the subseasonal forecast of low frequency rainfall over the lower reaches of Yangtze River Valley based on the 50-80 d oscillation [J]. Acta Meteorologica Sinica, 2016, 74(4): 491–509.
[8] Yang, Q. M. Datasets from the sub-seasonal forecast for the low frequency rainfall over the lower reaches of the Yangtze River Valley on the time scale of 50 to 80 d [DB/OL]. Global Change Research Data Publishing & Repository, 2018. DOI: 10.3974/geodb.2018.03.11.V1.
[9] GCdataPR Editorial Office. GCdataPR data sharing policy [OL]. DOI: 10.3974/dp.policy.2014.05 (Updated 2017).
[10] Kalnay, E., Kanamitsu, M., Kistler, R., et al. The NCEP/NCAR 40-year reanalysis project [J]. Bulletin of the American Meteorological Society, 1996, 77: 437–471.
[11] Mo, K. C. Adaptive filtering and prediction of intraseasonal oscillations [J]. Monthly Weather Review, 2001, 129: 802–817.